Extensive Research: The AI Giant's Big Next Step
Breaking Barriers in Language, Robotics, and Emotional Intelligence
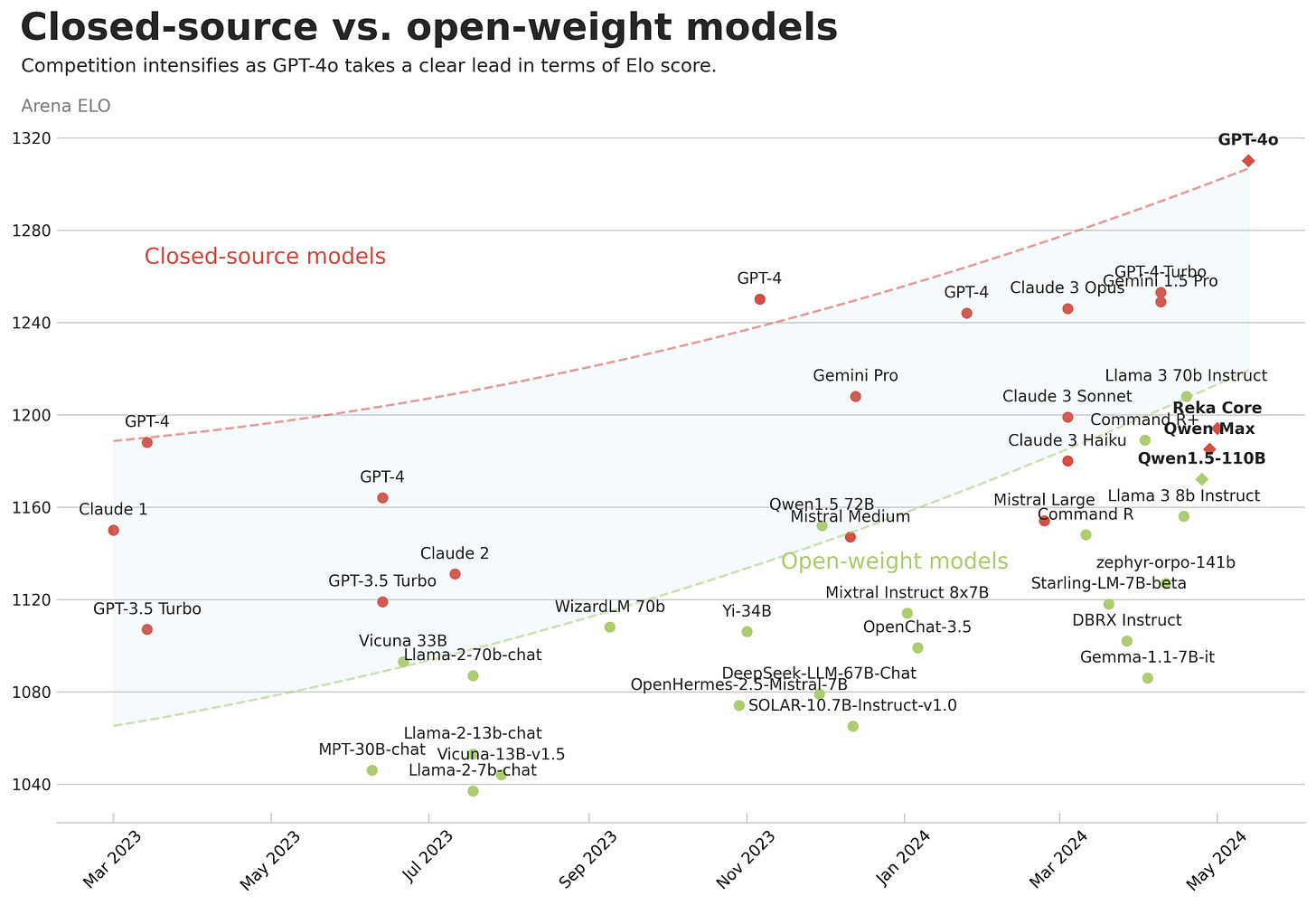
The Race for AI Supremacy
While closed-source models like GPT-4-Omni remain the pinnacle of language models, open-weight models are rapidly catching up, with recent releases demonstrating competitive performance. This trend signals a swift advancement in large language model (LLM) capabilities across the board. This AI arms race is playing out in real-time on live performance charts, making it a captivating space to watch.
The Next AI Frontier: Emotional Intelligence
Much of the AI sector is currently obsessed with making generative models bigger and more powerful by feeding them more data and compute power. However, the next significant leap in AI development may not come from sheer size but from teaching emotional intelligence to these models.
Current generative models lack the ability to understand and interpret human emotions effectively, a crucial aspect of successful communication. The next frontier in AI may, therefore, be enhancing these models with emotional intelligence.
This challenge has already captivated Mark Zuckerberg, who recently discussed the topic with podcaster Dwarkesh Patel. Zuckerberg highlighted the human brain's dedicated resources for understanding emotions and expressions, underscoring its importance as a distinct modality. Startups like Hume AI, attracting significant funding, are already making strides in this area by developing AI systems with emotional intelligence capabilities, hinting that emotionally aware AI may be on the horizon.
Robotics: Cracking the Physics Code
Marc Andreessen described the current state of robotics, where successful robots in manufacturing are typically rigidly programmed to perform very specific tasks with little adaptability.
A major roadblock to achieving sophisticated, adaptable robotics is the inability of current systems to truly understand the laws governing the physical world. While manufacturing robots excel at pre-programmed tasks, they struggle with flexibility and dynamic environments.
Two diverging theories have emerged to solve this puzzle:
The Data-Driven Approach: By exposing AI models to vast real-world datasets, they can implicitly learn and model the principles of physics, enabling accurate predictions and intuitive grasps of physical phenomena. This method is embodied by companies like Covariant.
Explicit Physical Modeling: Others argue inferring physics from data alone is insufficient. They propose developing new AI architectures that fundamentally encode an understanding of physics and 3D space from the ground up, as seen in Nvidia's Modulus project.
Despite taking contrasting philosophies, both camps are making steady progress, fueled by the industry-wide drive to push the boundaries of robotic capability.
The Power of the Data Flywheel
While the physics debate rages on, the transformative power of large datasets on AI capabilities is undeniable. From image recognition to self-driving cars and language models, data-driven approaches have proven remarkably successful in recent years.
This is exemplified by Tesla's strategy of treating its entire fleet as roving sensors, continuously gathering real-world training data to enhance its self-driving AI. This virtuous "data flywheel" allows the system's performance to rapidly compound over time.
However, the voracious appetite for data also raises regulatory concerns around privacy and consent. There are also open questions around addressing the long tail of edge cases and failure modes when dealing with safety-critical AI systems like autonomous vehicles.
The Road Ahead
As the AI field charges forward, key frontiers like embedding emotional intelligence, imbuing a nuanced understanding of physics, and thoughtfully harnessing the power of data will define the next generation of artificial intelligence. But ethical considerations around data privacy, bias, and responsible development must also be carefully navigated.
Major players like OpenAI are taking steps to increase transparency around these ethical challenges. Their recently released "Model Behavior Specification" document outlines their approach to shaping the desired behavior of language models through rules, principles for third-party developers, and guidelines for resolving conflicts between user inputs and model objectives. This provides a window into the decision-making processes that AI companies employ when deploying systems that can significantly impact society.
Initiatives like OpenAI's behavioral specifications demonstrate the AI industry's growing recognition of the importance of developing frameworks and standards to ensure artificial intelligence remains safe, aligned with human values, and ultimately benefits humanity. Complementing the relentless drive for technological advancement with robust governance will be paramount.
The race for supremacy is on, with the winners poised to build formidable and sustainable AI-driven competitive advantages. For the field's pioneers and paradigm-shifting companies, the future remains rife with both immense opportunity and profound challenges to thoughtfully overcome.
Reference
Chatbot Arena: An Open Platform for Evaluating LLMs by Human Preference
Introducing the Model Spec, Open-AI
AI & Robotics, Tesla
Hey there!
I'm Kevin Wang, a product manager by day and a passionate builder of tulsk.io, a Gen-AI Tool, in my free time. I'm on a mission to simplify complex concepts in web3 and AI, so they're easier to grasp and apply to your own learning and growth.
Keen to dive deeper?
Join the tulsk.io community and support my mission to share insights with budding entrepreneurs like you. Your support means the world to me and ensures I can continue to inspire innovation and knowledge.
๐ Spread the Curiosity! ๐
Share it to inspire innovation and knowledge!